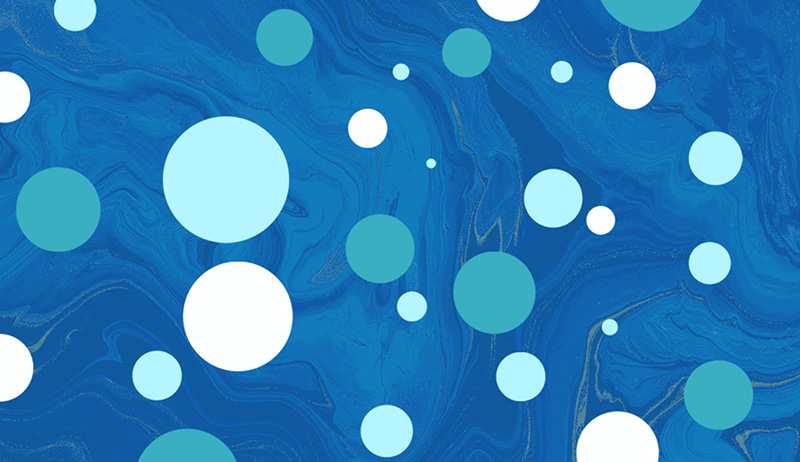
Most machine learning algorithms can’t learn from experiences beyond initial training. Now, MIT researchers have developed a type of neural network that learns on the job, not just during its training phase. These flexible algorithms, dubbed “liquid” networks, change their underlying equations to continuously adapt to new data inputs. This liquid neural network has a kind of built-in “neuroplasticity.” That is, as it goes about its work—say, in the future, maybe driving a car or directing a robot—it can learn from experience and adjust its connections on the fly.
Read more MIT Researchers Develop Skin-Like Device That Can Help ALS Patients Communicate
“This is a way forward for the future of robot control, natural language processing, video processing — any form of time series data processing,” says Ramin Hasani, the study’s lead author. “The potential is really significant.”
Hasani said the system is inspired by a tiny worm the C. elegans, which has 302 neurons in its nervous system yet it can generate unexpectedly complex dynamics.
The research will be presented at February’s AAAI Conference on Artificial Intelligence. In addition to Hasani, a postdoc in the MIT Computer Science and Artificial Intelligence Laboratory (CSAIL), MIT co-authors include Daniela Rus, CSAIL director and the Andrew and Erna Viterbi Professor of Electrical Engineering and Computer Science, and PhD student Alexander Amini. Other co-authors include Mathias Lechner of the Institute of Science and Technology Austria and Radu Grosu of the Vienna University of Technology, reports MIT News.
“The real world is all about sequences. Even our perception — you’re not perceiving images, you’re perceiving sequences of images,” Hasani says. “So, time series data actually create our reality.”
He points to video processing, financial data, and medical diagnostic applications as examples of time series that are central to society. The vicissitudes of these ever-changing data streams can be unpredictable. Yet analyzing these data in real-time, and using them to anticipate future behavior, can boost the development of emerging technologies like self-driving cars. So Hasani built an algorithm fit for the task.

Hasani says his liquid network skirts the inscrutability common to other neural networks. “Just changing the representation of a neuron,” which Hasani did with the differential equations, “you can really explore some degrees of complexity you couldn’t explore otherwise.” Thanks to Hasani’s small number of highly expressive neurons, it’s easier to peer into the “black box” of the network’s decision making and diagnose why the network made a certain characterization.
In tests, the network performed promisingly in predicting future values in datasets, ranging from atmospheric chemistry to traffic patterns.
Read more Scientists From NTU Singapore Develop AI System For High Precision Recognition of Hand Gestures
Hasani plans to keep improving the system and ready it for industrial application. “We have a provably more expressive neural network that is inspired by nature. But this is just the beginning of the process,” he says. “The obvious question is how do you extend this? We think this kind of network could be a key element of future intelligence systems.”